EyeVi Technologies is thrilled to announce that we have been chosen to participate in the DOT Studio, a partnership between the New York City Department of Transportation (NYC DOT) and Newlab. The DOT Studio supports early-stage ventures like us to build, test, and launch advanced technology solutions across the focus areas of electric vehicle charging and pavement marking analysis in New York City.
“The DOT Studio, a partnership between New York City Department of Transportation (NYC DOT) and Newlab, supports early-stage ventures in building, testing, and launching advanced technology solutions across the focus areas of EV Curbside Charging and Pavement Marking Analysis in New York City. The Studio aims to generate learnings and de-risk technology solutions to build a safe and sustainable transportation future.” - the DOT Studio
We are excited to have the opportunity to test our pavement marking solution in a real-world environment. This will allow us to see how well our system works in detecting road markings and identifying potential areas for improvement. We will also be able to evaluate different methods for understanding the condition of road markings. This will help us determine which approach is most effective in ensuring that our system can provide accurate and up-to-date information. We believe that this testing will provide valuable insights that will help us further improve our system.
Why New York and road markings?
The overall goal of the pilot is to develop a methodology for detecting and quantifying the wear of road markings by comparing aerial orthophotos over different time periods.
Road markings are essential to road safety, providing critical information to drivers about the road ahead. Unfortunately, road markings can be difficult to see, especially at night or in low light conditions. Our solution uses AI to automatically extract road markings from orthophotos, providing a clear and concise overview for road maintenance crews. This way, they can easily identify areas where road markings need to be repaired or replaced.
We will use AI to provide road marking information and their wear using high-resolution orthographic imagery. In this pilot, we will test our AI software to detect road markings and evaluate their wear based on orthophotos provided by NYC DOT.
We believe that this technology can help improve road safety and efficiency for DOT workers, New Yorkers, and tourists. Using our processed data, NYC DOT can maintain road markings in good condition.
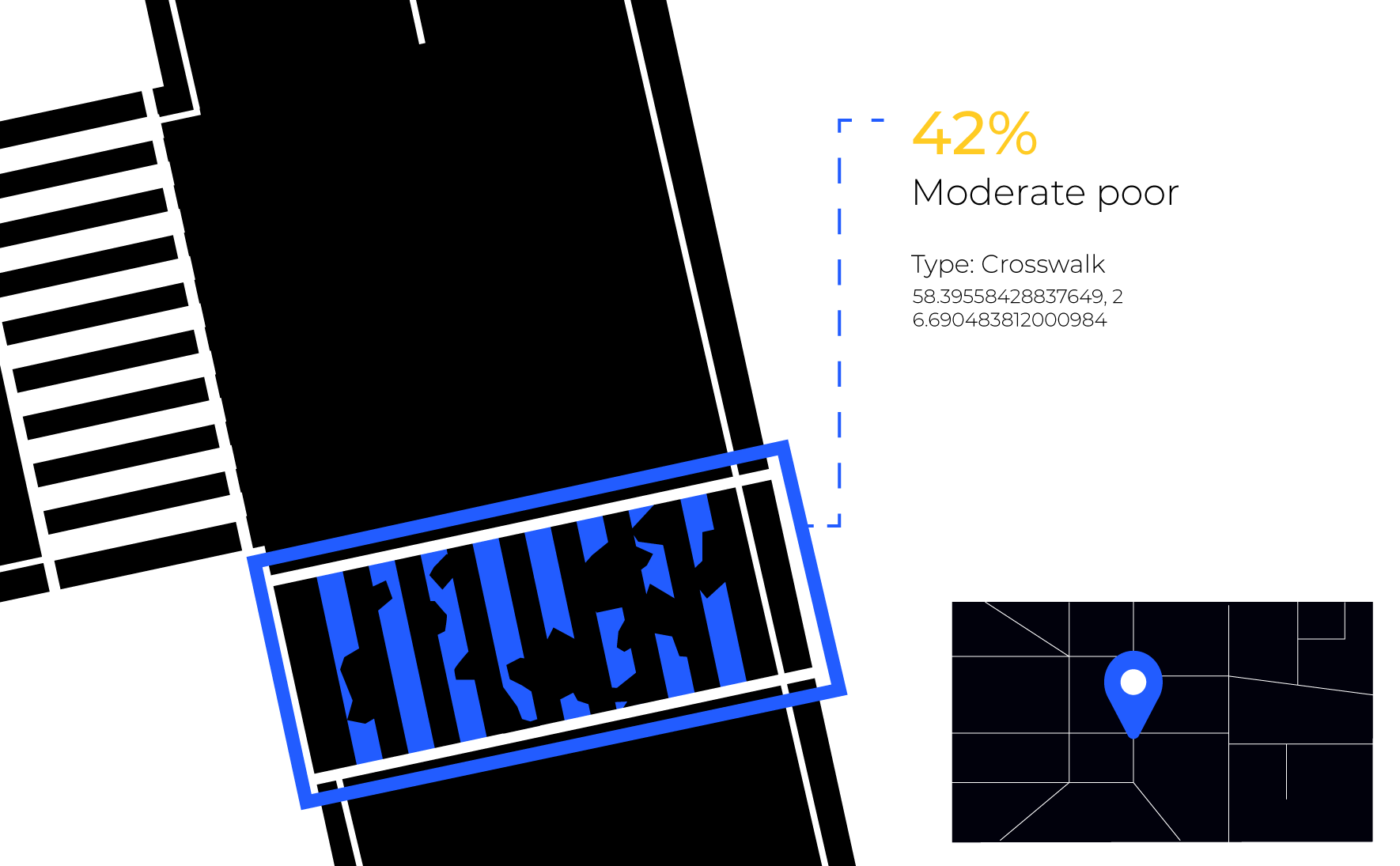
The challenges ahead
Any method used to evaluate the condition of road markings will need to account for traffic, parking, and vegetation.
In an ideal world, aerial photographs would provide sufficient information for both AI and non-AI road marking detection data extraction. They would be clear and consistent, and there would be no obstacles to block their view. However, the reality is that various real-world factors can make it difficult for AI to properly recognize street markings and this can cause inaccurate or inconsistent analysis. For example, shadows, debris, and obstacles like cars, buildings, and trees can obscure the view.
If a car is parked in the same spot over a period of time, the road markings in that area will appear to change due to wear. However, if the car is moved or the area is cleaned, the road markings will appear different in the new image. As such, it is essential to account for these local effects when monitoring road markings for changes over time.
AI-powered software must rely on multiple data sets and consistently updated algorithms to interpret the environment in question. As EyeVi’s AI continues to learn, adapt and improve, it is our goal to resolve these issues that have previously hampered AI solutions to road infrastructure safety.
How EyeVi and Newlab will rise above
How do we plan to overcome these challenges?
Our first task is the evaluation of aerial orthophotos and there are a few key factors to keep in mind. First, we will evaluate the image quality. This includes things like resolution and coverage. Next, we’ll look at the geolocation accuracy. This is important because it can impact variables such as traffic and parked cars. We will also consider factors that may have impacted the data capture conditions, such as weather and light conditions.
By taking all these factors into account, we aim to get the most information out of the aerial orthophotos and determine how and to what extent they can be used.

We will test both AI and non-AI approaches for the second task of detecting road markings from images. We will begin by testing our pre-existing AI road marking detection algorithms. These algorithms were originally developed for EyeVi MMS (Mobile Mapping System) orthophotos.
Aerial orthophotos are slightly different because they are not as high resolution and have more comprehensive coverage (MMS orthophotos cover only the road network, aerial orthophotos cover everything in between as well). The aim is to find which approach works better on our data set and under what conditions.
The third task is the classification of road markings. This is crucial to understanding wear and tear. By classifying markings based on color and type, we can better analyze which markings are more susceptible to deterioration. This, in turn, helps road maintenance crews make better decisions about maintenance. For example, if we find that only crosswalks and longitudinal markings show signs of wear, then maintenance crews can focus their efforts on those specific types of markings.
Conversely, if different types of markings show varying signs of wear, then maintenance can prioritize their work accordingly. In either case, the classification of road markings is a vital step in ensuring that the right decisions are made about maintenance.
Along with type and color classification, a wear condition class is assigned to road markings. This ranges from good to very poor and is based on how much of the road marking remains intact. Based on that, an action can be decided to refurbish the road markings or not.
We will also attempt to detect the condition of road markings by comparing datasets between fall and spring – specifically the geometric changes to road markings. Of course, this kind of analysis is highly sensitive to obstructions such as cars, vegetation, dirt, buildings, etc.
That is why we will detect these as well or to put it another way - detect the road area without any obstructions. In that case, only areas where the road surface is visible on both images will be included in the analysis.
As usual, the AI detection and classification steps are refined by several iterations of annotation and AI training. The AI results can be used as a base to make the annotation quicker and easier.
Finally, all the results will be linked to the LION geographic base file of New York City streets. This means that they are usable in regular NYC DOT workflows.
By comparing the condition of road markings between fall and spring, we can detect areas where the markings are wearing down more quickly. This information can then be used to make repairs or take other preventive measures to extend the life of the road markings.
What’s to gain from road markings?
The scope of the project is quite large and a great step forward for EyeVi, because the research gathered, the application to our underlying AI-powered solutions, and the real-world applications will directly benefit the New York City Department of Transportation and many more large municipalities to come.
The detection of road markings and their condition is just one piece of what EyeVi does. We are excited to be part of a project that represents the relentless pursuit of our vision of the future of infrastructure maintenance: exponentially increased efficiency, reduced road maintenance costs, and optimized prioritization of road work. Ultimately, the real-world value will result in improved safety for drivers and pedestrians alike.
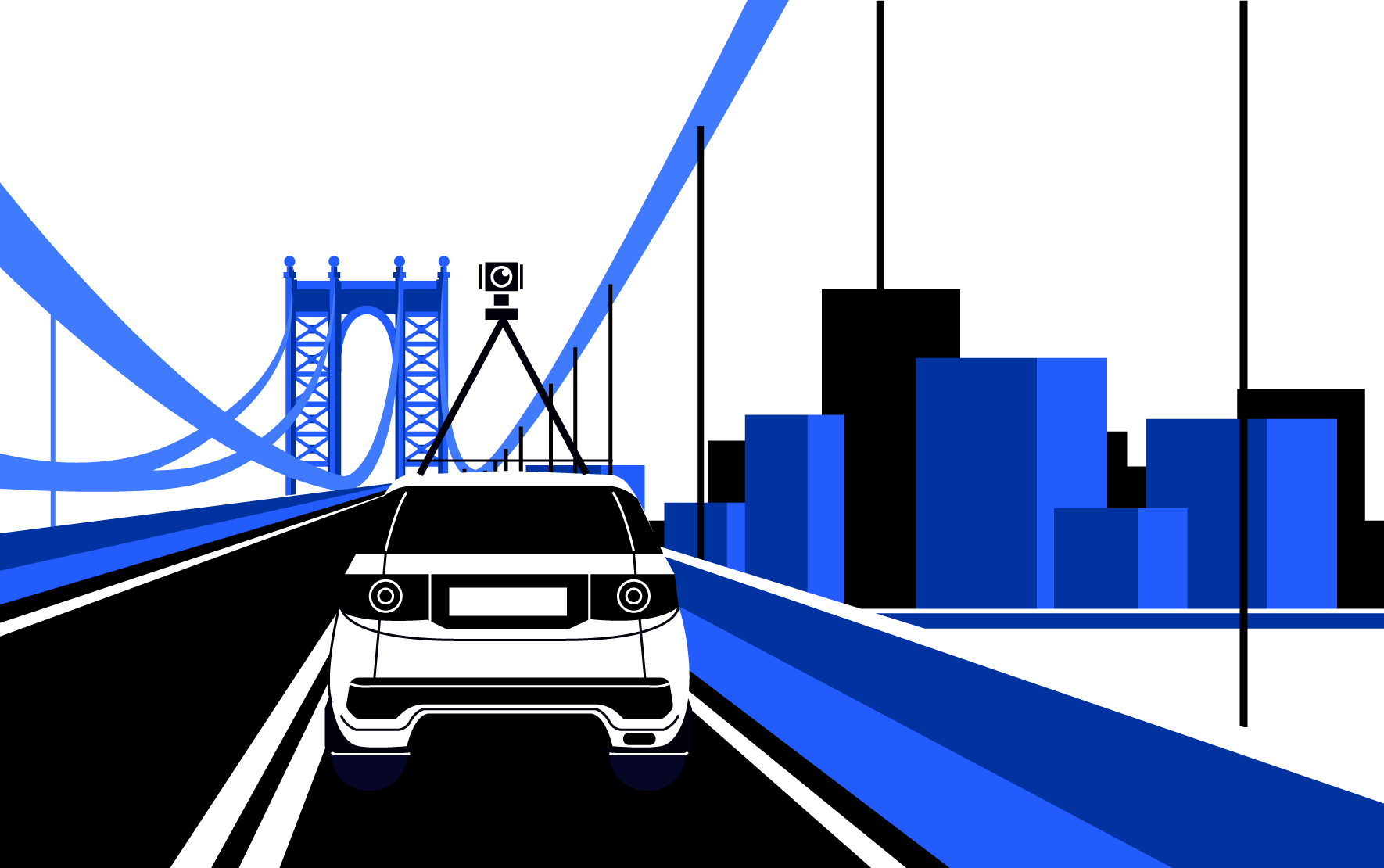